"我读博士的期间,有幸有三位人工智能的图灵奖得主,分别指导我的科研。其中一位就是最新的图灵奖得主Geoff Hinton。下面是提名辛顿时,我写给ACM评委的推荐信:"---by 李开复
李根 唐旭 发自 凹非寺 量子位 报道 | 公众号 QbitAI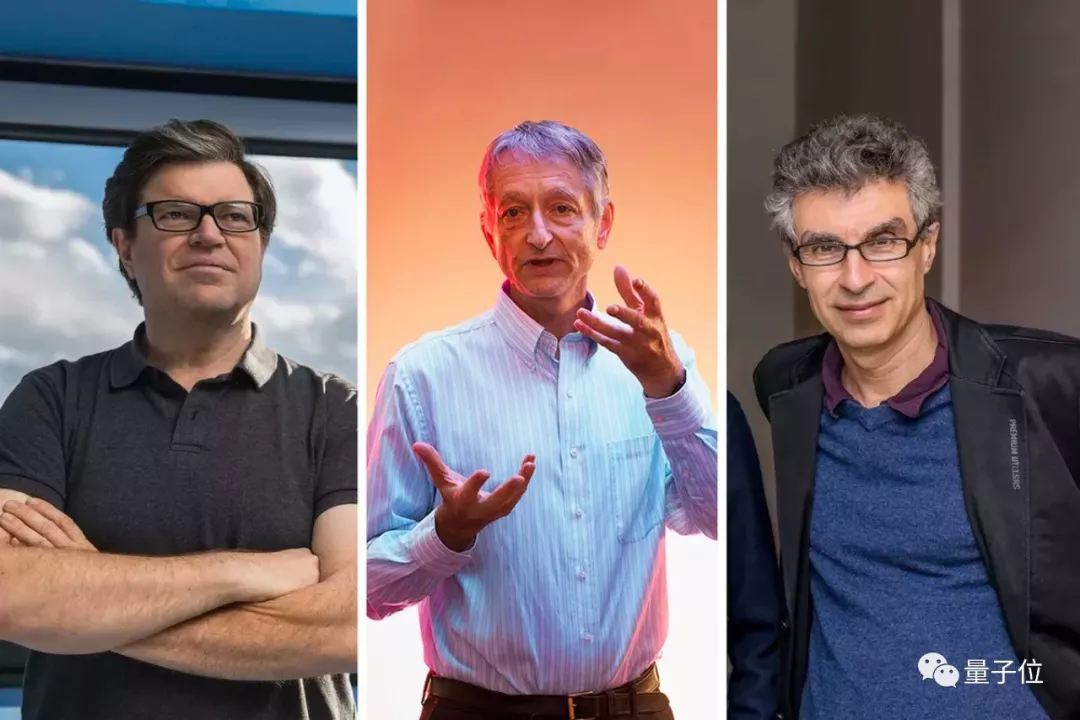
3月27日,2018年图灵奖嘉奖正式揭晓:深度学习三巨头Yoshua Bengio、Geoffrey Hinton,Yann LeCun一起荣膺计算机领域的最高荣誉。
ACM评委会颁奖词称:表彰他们以概念和工程的突破,让深度神经网络成为计算关键部件。 其中,72岁的Geoffrey Hinton更是众望所归,在年盛一年的呼声之后,终于加冕图灵奖。 评选揭晓后,量子位获悉,其实今年全球多位AI领域影响力科学家,就已经提名Geoffrey Hinton且撰写了推荐信。 按照图灵奖提名推荐流程,推荐人须与被推荐者曾经共事。 所以包括Google研究负责人Jeff Dean、创新工场董事长李开复、微软研究院掌舵者Eric Horvitz,以及此次一同获奖的Yann LeCun等,都因曾经共事而提名了Geoffery Hinton。 现在,我们获得授权,披露李开复向ACM图灵奖评选委员会提名Geoff Hinton的推荐信。 需要说明的是,原件为英文,量子位进行了编译。 但强烈推荐学有余力的盆友直接读英文原文(后附),一方面是因为李开复围绕学术、产业和教育等三大方面,对Geoffrey Hinton进行功绩介绍,字里行间也不乏一些鲜为人知的小故事,还有溢于言表的钦佩和赞美。 另一方面,也确实锻炼英文的好机会。 OK,Here we go~ 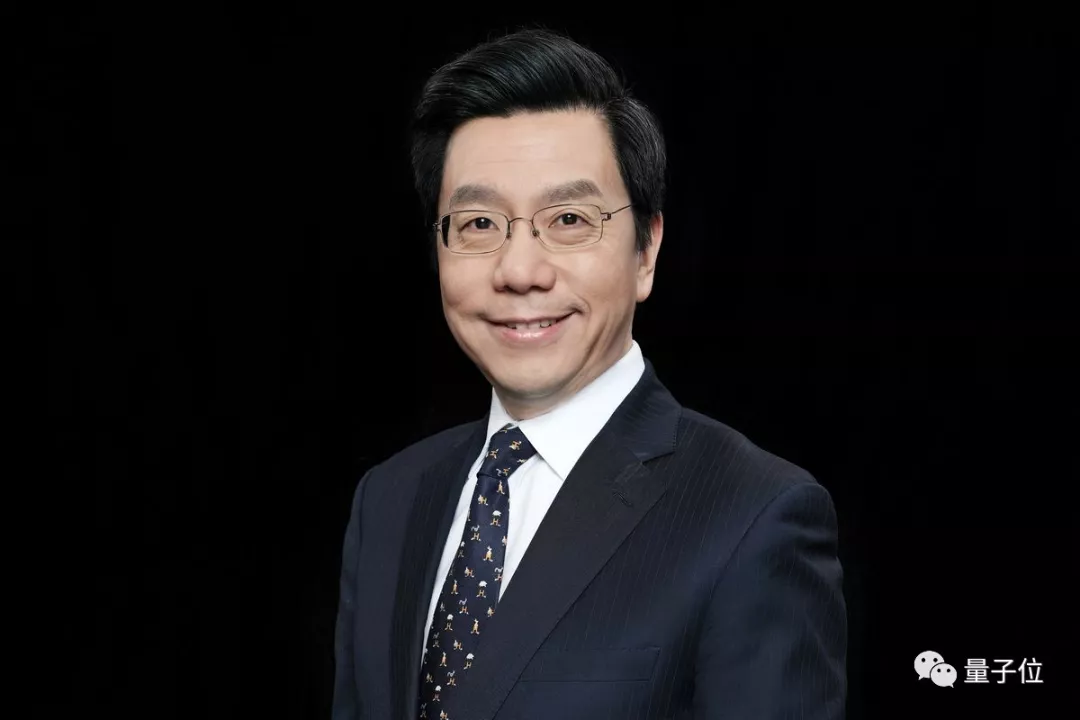
李开复推荐信(量子位编译版)尊敬的ACM图灵奖评选委员会: 我谨以此文表达个人最强烈的推荐和支持,提名Geoff Hinton为本年度的图灵奖候选人。这是人工智能的时代,而在人工智能领域,没人能比Geoff更有资格获得这份嘉奖。 我现在是创新工场董事长及CEO,并曾作为高管任职于苹果、SGI、微软和谷歌。我曾是卡内基梅隆大学的助理教授,同时在这所大学获得了博士学位。 也正是在CMU,1983年,我作为一名博士生结识了Geoff:修读了他的神经网络课程;并和他的研究团队一起,尝试将神经网络应用于语音识别;我还在他的指导下完成了自己的辅修课程论文(关于将贝叶斯学习应用于博弈游戏),我的博士论文(用机器学习方法进行语音识别),也曾向Geoff和他的团队寻求过建议。 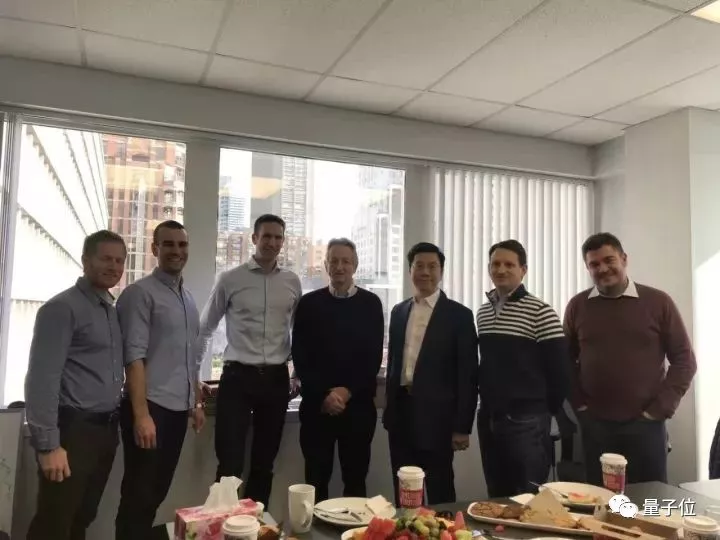
△Hinton和李开复2017年会面,量子位配图
虽然Geoff并非我的博士生导师,但他对于我博士论文的影响却十分巨大。他的学生Peter Brown(统计机器翻译的共同发明人,如今文艺复兴科技的CEO)当时是我的老师,也正是他教会了我如何把不同种类的机器学习算法应用于语音识别,为我的博士论文打下了基础。 其后1988年,我的博士论文实现了当时最好的语音识别模型,也促使语音识别领域,将重心从专家系统方法转移到机器学习方法。鉴于Geoff的才华、坚韧和慷慨,如果我能从Geoff和Peter身上受益如此之多,想必还有成千上万的受益者跟我一样。 Geoff对于AI领域的贡献是巨大、无可比拟的。在我最近出版的畅销书 AI Supowerpowers: China, Silicon Valley, and the New World Order(中文名《AI·未来》)中,以通俗的说法描述了Geoff对于AI领域的贡献: 曾经,人工神经网络能做的事非常有限。复杂问题若要得出准确的结果,必须构建很多层的人工神经元,但神经元层数增加后,研究人员当时还未找到针对新增神经元有效的训练方法。 在21世纪头10年的中期,深度学习这项重大的技术性突破终于到来,知名研究人员杰弗里·辛顿找到了有效训练人工神经网络中新增神经元层的方法。这就像是给旧的神经网络打了一针兴奋剂,使它们的能力倍增,可以执行更多、更复杂的工作,例如语音及物体识别。 性能大增的人工神经网络——现在有了新的名字“深度学习”——开始把旧的系统远远甩在身后。多年来对神经网络根深蒂固的成见让人工智能的许多研究人员忽略了这个已经取得出色成果的“边缘群体”,但2012年杰弗里·辛顿的团队在一场国际计算机视觉竞赛中的胜出[1],让人工神经网络和深度学习重新回到聚光灯下。 在边缘地带煎熬了数十年后,以深度学习的形式再次回到公众视野中的神经网络法不仅成功地让人工智能回暖,也第一次把人工智能真正地应用在现实世界中。研究人员、未来学家、科技公司CEO都开始讨论人工智能的巨大潜力:识别人类语言、翻译文件、识别图像、预测消费者行为、辨别欺诈行为、批准贷款、帮助机器人“看”,甚至开车。
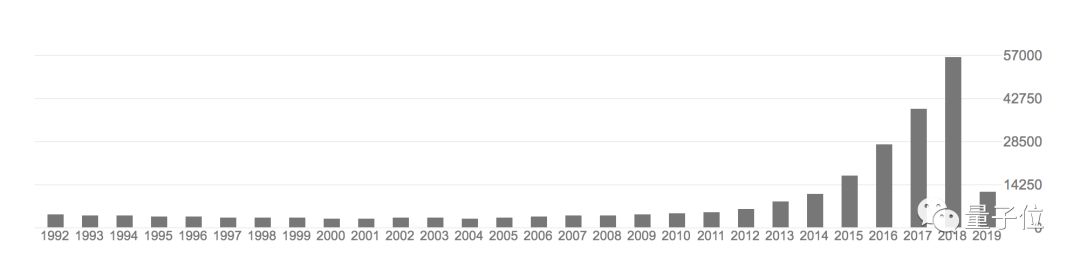
如果就学术成果而言,Geoff的引用超过25万次,其中一半以上来自于过去5年;他的H指数是惊人的142;他是波茨曼机以及通过梯度下降法实现反向传播(前者与Terry
Sejnowski共同发布于1983年,后者则在1986年与David
Rumelhart共同发布于《自然》杂志)两项开创性工作的共同发明人,这些工作引入了神经网络中隐藏层的思想,以及一种用于对其附属参数进行训练的优美、易于计算的方法。
隐藏层让软件从“人类控制“(如专家系统)下得到解放,而反向传播则让非线性组合系统发现突出特征成为可能,以一种更接近于目标而非人类驱动的方式。 然而,这些思想对于其所处时代而言,当时过于超前。因为当时并没有足够的数据和算力,能让这些理论上的方法解决实际生活中的问题,或是在竞争中压倒其他方法。 于是在20世纪80年代初期,该领域被专家系统所统治,直到80年代末期,专家系统因过于脆弱和难以扩展成为历史。 不过替代专家系统的也并非Geoff提出的构想(那时还太早),而是妥协于少量数据和算力的简化版本的神经网络。 我的博士论文(使用了隐马尔科夫模型)讨论的就是其中的一种。这些简化过的方法确实能在一部分应用上作出贡献,但如同专家系统一样,它们并不能在那些最难解决的问题上进行扩展,如下围棋、人类水平的语音和视觉。 然而时来运转,从1985年到2015年,全球数据量和算力发生了巨大的增长。 举例来说,我在1988年的博士论文使用了当时最大的语音数据库,但它也只有100MB的大小。 而今天,最好的语音识别系统要在100TB的数据上进行训练——这是一百万倍的提升。有了数据上如此巨大的提升作为支持,Geoff的思想最终闪耀了起来——他的方法能将层的数量由1增加到1000,而数据量和模型复杂度的提升会使得深度学习系统持续进步。 事后再看这些问题,显得异常容易。但在当时,现实却是非常残酷的。20世纪90年代对于像Geoff一样的神经网络研究者而言,是最黑暗的时刻。 Geoff早期的理论工作创造出了智能的火花,但数据和算力的匮乏却阻碍了这些深度学习系统展示出更优秀的性能。随着科研经费消耗殆尽,许多神经网络研究者将自己的工作转移到了其他领域。然而,面对黯淡而又浮躁的科研资助环境,Geoff依然作为少数研究者(其他关键性研究者包括Yann
LeCun和Yoshua Bengio)坚持了下来,不懈地将神经网络方法继续向前推进。 他搬到了加拿大,根据受限的经费环境调整了团队,而后继续努力将科学的边界向前拓展。 Geoff在接下来的30年中持续地为神经网络方法做着贡献,其中包括多专家模型、亥姆霍兹机、神经动画生成系统、概率推理模型、随机领域嵌入、邻域组件分析、t-SNE,以及诸多创新思想和研究。 鲜有某项技术可以彻底颠覆多个领域的研究,而深度学习就是其中之一。从2010年到2016年,本质上讲,整个感知领域——语音识别、图像识别、计算机视觉都转移到了深度学习的路径上,就是因为Geoff和他的同事们证明了——对于感知而言,深度学习就是最佳也最能普及的方法。 在整个人工智能领域,人类的感知能力(听、看和理解)被视作人类独有的天赋,但对于AI而言,这是一个巨大挑战。值得一提的是,还有一项巨大的挑战是下围棋,已经被Deepmind开发的AlphaGo,同样使用深度学习方法攻克了。当时震惊了整个世界,也成了“AI革命”的催化剂。 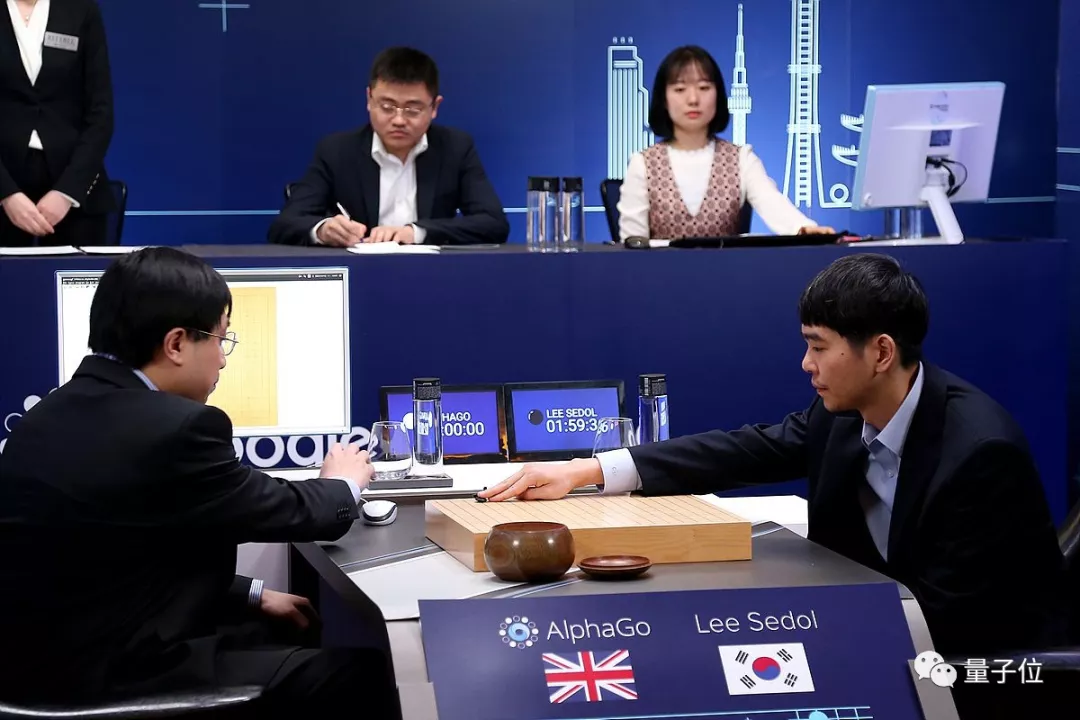
现在回顾,Geoff的团队是如此颠覆计算机视觉研究的:2012年,他的团队构建了一个基于神经网络的系统,在ImageNet 1000个类的物体识别竞赛中将错误率一下降低了40%。
在此之前,计算机视觉领域的研究社群已经习惯了每年一小部分的增量提升,而Geoff团队的成绩震惊了整个社群——人们未曾想象过,一个“局外人”会以一种“非传统方法”以如此大的优势赢下竞赛。 如果说反向传播是Geoff最重要的理论贡献,那么其团队在ImageNet竞赛中取得的成果则是Geoff最为人认可的贡献。那届ImageNet竞赛结果所掀起的微波,最终成为了深度学习大潮中的滔天巨浪。 深度学习的浪潮如今也正在改变每一个行业。举例来说,作为一名身处中国的投资人,我本人也品尝过这股春风带来的甘霖:Geoff在2012年发表的论文以及当年ImageNet上的成果,为4家中国的计算机视觉公司带来了灵感,而现在他们的总估值超过120亿美元。但请记住,这还只是Geoff的工作在一个国家、一个小小的领域内带来的成果。 此外,Geoff的工作还使得深度学习颠覆了语音识别领域(也是我博士时期的研究方向),帮助当时加盟百度的吴恩达,在2015年使得机器识别的准确度超越人类。 从更广阔的视角上看,世界上的每一家科技巨头(谷歌、微软、IBM、Facebook、亚马逊、百度、腾讯和阿里巴巴)都为深度学习打造了自己的平台,甚至将自己重新标榜为“AI公司”;而在风投领域,我们则见证了深度学习驱动下大批独角兽公司的出现(仅在中国就有超过20家)。 同时,由于深度学习需要的强大算力无法从传统的CPU中获得,为了负载深度学习所需的工作量,GPU开始被大规模使用,并由此引发了英伟达的崛起以及半导体工业的复活。 最为重要的是,我们的生活已经发生了深刻的变化:从搜索引擎,到社交网络再到电子商务,从无人商店到无人汽车,从金融到医疗,几乎所有能想象到的领域,都被深度学习的力量所重塑,或是颠覆。 在任何具有充足数据的领域,深度学习在用户体验、用户粘性、营收和利润方面都带来了极大的提升。深度学习背后的核心思想(始于反向传播)——一个目标函数可以被用来使商业指标最大化——已经对所有行业造成了深刻的影响,并帮助那些拥有数据、拥抱机器学习的公司获得了难以置信的利润。 总的说来,人工智能可以说是当今我们能够成熟应用的技术中,最为令人兴奋的一种。普华永道和麦肯锡预计,在2030年以前,AI会给全球GDP带来12到16万亿美元的增长。 而AI领域最重要的进展,以及AI技术的成熟度被坚信的首要原因,就是Geoff在深度学习方面的工作成果。 诚然,每一位图灵奖的获奖者都对计算机科学领域有着极其重大的影响,但极少数能像Geoff一样,改变了整个世界。 在变革者的角色之外,Geoff还是一位真正的思想领袖。虽然他总是言辞温和,他却是一位真正塑造并重塑整个研究社群的精神领袖。 他不知疲倦地教诲,不只是对他的学生,更是对这个世界。 正如他1986年创办联结主义学院时,亲自与在计算机视觉和语音处理领域的人们去进行沟通,说服他们去理解并拥抱机器学习。然而,当2018年所有的工作都获得了成功,整个世界都投入了深度学习的怀抱之时,他还继续指出一条全新的道路。 在行业纷纷向深度学习靠拢,大公司们不断收集更多的数据并开始引领深度学习的“工业化”之时,他却号召人们向前一步,去创造“下一个深度学习”。换而言之,解决AI根本问题的一种全新方法,帮助机器更接近真正的人类智慧。 他在思想上的领袖魅力来源于他毕生的愿景,以及对于更好地去理解人类认知能力的追寻。尽管深度学习是一项正在改变世界的重大突破,他却仅仅将其视为实现自己长期愿景路途中的一块踏脚石。他最近在胶囊网络方面的新工作,也正再一次让研究者们重新审视自身在Geoff愿景中的角色和责任。 我坚信,Geoff就是今天的人工智能领域内最重要的人物,没有之一。 他对于学界和业界的贡献同样地突出。他不仅是一位优秀的、引领性的学者,亦是一位孜孜以求、慷慨、坚韧、优雅、有原则的绅士。他是所有年轻计算机科学家的楷模。他的工作大大超越了神经网络和机器学习的范畴,极大地影响了计算机视觉、语音及信号处理、统计学、认知科学以及神经科学。 我想不出其他任何人比他更有资格获得图灵奖,并在此敦促评选委员会在今年选择Geoff作为获奖人。 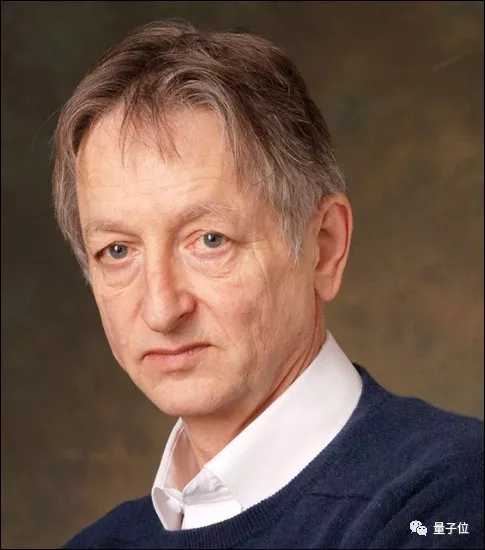
英文原件内容Dear ACM Turing Award Committee Members: I
am writing to give my strongest recommendation to support Geoff
Hinton’s nomination for Turing Award. This is the decade of Artificial
Intelligence, and there is no one more qualified than Geoff in AI. I
am the Chairman and CEO of Sinovation Ventures, and have previously
held executive positions at Apple, Microsoft, SGI, and Google. I was an
assistant professor at Carnegie Mellon, and also received my Ph.D.
there. I got to know Geoff at Carnegie Mellon, when I entered as a Ph.D.
student in 1983. I took classes on neural networks from him, worked
with his research team on applying neural networks to speech
recognition, and was supervised by him for my minor thesis (on applying
Bayesian learning to game-playing), and consulted him and his team on my
Ph.D. thesis (machine learning approach to speech recognition). While
Geoff was not my Ph.D. advisor, his impact on my Ph.D. thesis was
tremendous. His student Peter Brown (co-inventor of statistical machine
translation, now CEO of Renaissance Technologies) was my mentor, and
taught me how to apply various types of machine learning algorithms to
speech recognition. This was a primary reason that helped my Ph.D.
thesis to become the best-performing speech recognizer in 1988, which
helped shift the speech recognition field from expert-systems approach
to machine-learning approach. If I have benefited so much from Geoff and
Peter, there must be thousands of other beneficiaries, given Geoff’s
brilliance, persistence, and generosity. Geoff’s
contributions to AI are immense and incomparable. In my recent
best-selling book AI Supowerpowers: China, Silicon Valley, and the New
World Order, I described Geoff’s contribution as follows, in layman’s
language: Deep
learning’s big technical break finally arrived in the mid-2000s, when
leading researcher Geoffrey Hinton discovered a way to efficiently train
those new layers in neural networks. The result was like giving
steroids to the old neural networks, multiplying their power to perform
tasks such as speech and object recognition. Soon,
these juiced-up neural networks—now rebranded as “deep learning”—could
outperform older models at a variety of tasks. But years of ingrained
prejudice against the neural networks approach led many AI researchers
to overlook this “fringe” group that claimed outstanding results. The
turning point came in 2012, when a neural network built by Hinton’s team
demolished the competition in an international computer vision contest. In
the twelve years since Geoffrey Hinton and his colleagues’ landmark
paper on deep learning, I haven’t seen anything that represents a
similar sea change in machine intelligence. In
terms of academic accomplishments, Geoff has more than 250,000
citations, with more than half in the last five years. He has an
astoundingly high H-index of 142. He was the co-inventor of the seminal
work on Boltzmann Machines and backpropagation using gradient descent
(published in 1983 with Terry Sejnowski, and the Nature paper with David
Rumelhart in 1986). This work introduced the idea of hidden layers in
neural networks, along with a mathematically elegant and computational
tractable way to train their affiliated parameters. Hidden layers freed
the software from “human control” (such as expert systems) and back
propagation allowed non-linear combination to essentially discover
prominent features (in a more goal-directed way than humans) in the
process. However, it turned out that these ideas were before their time,
as there were not enough data or computing power to enable these
theoretical approaches to solve real-world problems or beat other
approaches in competitions. The early-1980’s were dominated by expert
systems, which became discredited in by late-1980’s when they were
proven to be brittle and unscalable. What displaced expert systems was
not Geoff’s proposals (which were too early), but simplified versions of
neural networks which were compromised to work with less data and
computation. My Ph.D. thesis (using hidden Markov models) was among
them, and these simplified approaches were able to make some
contributions with some applications, but like expert systems, they were
not able to scale to the hardest problems (such as playing Go,
human-level speech or vision). From
1985 to 2015, the amount of data and computation increased
tremendously. For example, my 1988 Ph.D. thesis used the largest speech
database at the time, which was only 100 MB. Today, the best speech
recognition systems are trained on 100 TB of data – a million-fold
increase. And with that much increase in data size, Geoff’s approach
(later re-branded deep learning) finally shined, as it could increase
the number of layers from one to thousands, and deep learning systems
continued to improve as the data size and the complexity of the model
increased. This
is easy to see in hind sight. But at the time, the reality was quite
cruel. The 1990s were the darkest hours for neural network researchers
like Geoff. Geoff’s earlier theoretical work created intellectual spark,
but the lack of data and computation prevented these deep learning
systems from demonstrating superior performance. Funding dried up, and
many neural network researchers moved away to other areas. But Geoff was
among the few researchers (other key researchers include Yann LeCun and
Yoshua Bengio) who persisted on pushing forward the neural network
approach, despite a frosty and fickle environment for funding. He moved
to Canada, adjusted his group to a smaller funding environment, and
continued to push the frontier. His
contribution to the neural network approach continued in the next 30
years, including the mixture of experts model, the Helmholtz machine,
the neural-animator system, probabilistic inference, stochastic neighbor
embedding and neighborhood component analysis, t-SNE, and many other
innovative ideas. Very
few technologies have disrupted multiple areas of research completely,
but deep learning did. From 2010 to 2016, essentially the entire field
of perception – speech recognition, image recognition, computer vision,
switched to deep learning, as Geoff and his colleagues proved deep
learning to be the best and most generalizable approach for perception.
In the entire field of Artificial Intelligence, human perception (to
hear, see, and understand) was considered one of the aspects that set
the humans apart and a grand challenge for AI (incidentally, playing Go
was another, which was conquered by Deepmind’s AlphaGo, which also used
deep learning during the matches which shocked the world, and was
another catalyst for the “AI revolution”). Here
is how Geoff’s team disrupted computer vision research. In 2012, his
team built a neural-network based system that cut the error rate by 40%
on ImageNet’s 1000-object recognition task and competition. The computer
vision community was accustomed to incremental improvements annually.
Geoff’s team’s results shocked that community, as a relative “outsider”
using an “unconventional approach” won by such a large margin. If
backpropagation was Geoff’s most important theoretical contribution, his
team’s work on the ImageNet competition was Geoff’s most recognized
contribution. That ImageNet result started the first ripple that
ultimately became the deep learning tidal wave. The
deep learning tidal wave (the most central part of the “AI revolution”)
is now changing every industry. As an example, as a venture capitalist
in China, I was a part of a “tiny” side effect: Geoff’s 2012 paper and
ImageNet result inspired four computer vision companies in China, and
today they are collectively worth about $12 billion. Keep in mind, this
was just one small field in one country based on one of Geoff’s result.
Geoff’s result also led to deep learning disrupting speech recognition
(the area of my Ph.D. work), resulting in super-human accuracy in 2015
by Baidu’s Andrew Ng (recruited to Baidu after Geoff joined Google
part-time). And much more broadly, every technology monolith (Google,
Microsoft, IBM, Facebook, Amazon, Baidu, Tencent, Alibaba) built its
platform for deep learning, and re-branding themselves as “AI
companies”. And in venture capital, we saw the emergence of many
unicorns (in China alone there are over twenty) powered by deep
learning. Also, deep learning required much compute power that
traditional CPUs could not handle, which led to the use of GPUs, the
rise of Nvidia and the re-emergence of semiconductors to handle deep
learning work-load. Most importantly, our lives have changed profoundly –
from search engines to social networks to e-commerce, from autonomous
stores to autonomous vehicles, from finance to healthcare, almost every
imaginable domain is either being re-invented or disrupted by the power
of machine learning. In any domain with sufficient data, deep learning
has led to large improvements in user satisfaction, user retention,
revenue, and profit. The central idea behind deep learning (and
originally from backpropagation) that an objective function could be
used to maximize business metrics has had profound impact on all
businesses, and helped the companies that have data and embraced machine
learning to become incredibly profitable. In
aggregate, Artificial Intelligence (AI) is arguably the most exciting
technology ripe for applications today. PWC and McKinsey predicted that
AI would add $12-16 trillion to the global GDP by 2030. The most
important advance and the primary reason that AI is believed to have
matured is Geoff’s work on deep learning. While every Turing Award
recipient has made seminal impact to Computer Science, few have changed
the world as Geoff is doing. Beyond
the role of an innovator, Geoff was also a true thought leader. While
he is soft-spoken, he is a spiritual leader who really shapes and
reshapes the overall research community. He was a tireless in teaching
not only his students but the world. For example, he started the
Connectionist School in 1986. He personally connected to and persuaded
people in computer vision and speech processing to understand and
embrace deep learning. Yet, after all that work succeeded, and the world
was won over by deep learning in 2018, he set a new direction. Because
industry has rallied around deep learning, and large companies were
gathering more data and leading the “industrialization” of deep
learning, he made an exhortation to move on and focus on inventing “the
next deep learning”, or fundamentally new approach to AI problems that
could move closer to true human intelligence. His
thought leadership was grounded in his life-long vision and quest to
better understand human cognition. While deep learning is a breakthrough
that is changing the world, he sees it as only a stepping stone towards
the realization of his long-term vision. To set another example, his
new work on capsule learning is again causing researchers to rethink
their role and responsibilities in Geoff’s vision. I
believe Geoff is the single most important figure in the field of
Artificial Intelligence today. His contributions to academia and
industry are equally outstanding. He is not only a brilliant and
inspirational scholar, but also an inquisitive, generous, persistent,
decent, and principled gentleman, who is a role model for any aspiring
young computer scientist. His work went well beyond neural networks and
machine learning, and has greatly impacted computer vision, speech and
signal processing, statistics, cognitive science, and neural science. I
cannot think of anyone else more deserving of the Turing Award, and urge
the committee to select Geoff as the recipient this year.
Sincerely, Kai-Fu Lee, Ph.D. Chairman & CEO, Sinovation Ventures Fellow, IEEE Honorary Ph.D., Carnegie Mellon University Honorary Ph.D., City University of Hong Kong
|